Remember when talking to a computer meant typing commands or going through confusing menus? Those days feel like ancient history now. We live in an era where our devices don’t just respond to us—they understand, chat with, and sometimes even anticipate what we need before we ask.
Conversational AI made this possible and transformed our interactions with technology, from virtual assistants managing schedules to chatbots resolving customer queries quickly. According to recent market analyses, the global Conversational AI market was valued at approximately USD 13.6 billion in 2024 and is projected to reach USD 151.6 billion by 2033, exhibiting a CAGR of 29.16% during the forecast period.
Behind these impressive stats is a blend of technologies: natural language processing (NLP) that deciphers messy human communication and machine learning algorithms that improve each conversation. Additionally, advanced dialogue management systems keep interactions flowing naturally.
In this deep dive, we will explore conversational AI. We will understand what makes an AI system “conversational” and how it differs from other AI approaches you might have heard about. We will also discuss how these systems work and the practical implementation of these technologies, including the platforms and tools available.
What is Conversational AI?
Conversational AI enables machines to understand and respond to human language, whether spoken or written, in a way that mimics natural human conversation. It’s about creating interactive experiences where users can communicate with computers using everyday language.
Think of it as the difference between a waiter who just takes your order verbatim vs. one who understands what you are in the mood for and can make recommendations based on your preferences and previous visits. One is transactional; the other creates an experience.
Here’s an overview of the features that enable Conversational AI:
- Understanding User Intent: Conversational AI systems can understand what users want or need from their input. Instead of relying on rigid commands, it aims to understand the underlying meaning behind the words.
- Context: Conversational AI aims to retain context during interactions, recalling past exchanges to deliver relevant and coherent responses.
- Processing Natural Language: These systems Use Natural Language Processing (NLP) to interpret and analyze human language, accommodating various dialects, slang, and colloquialisms.
- Natural Language Generation (NLG): This is the process by which the machine generates text in human-readable languages. After understanding the user’s input, NLG enables virtual agents to construct coherent and contextually appropriate responses in a clear and natural manner.
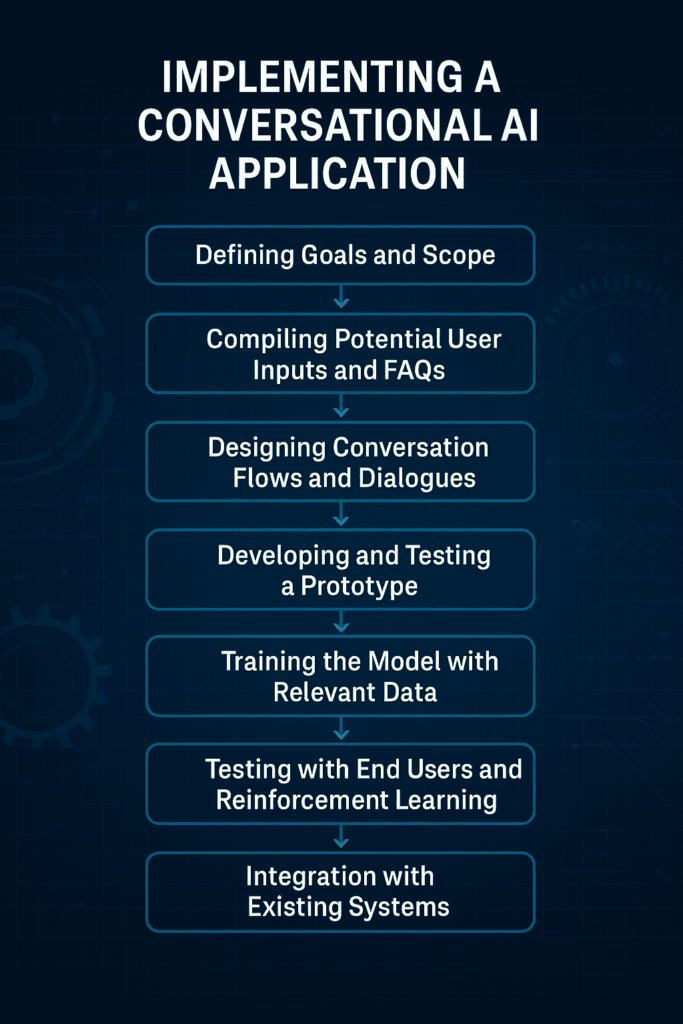
Benefits of Conversational AI
Conversational AI offers many advantages for businesses and their customers, impacting various operations and interactions. Here are some key benefits:
- Enhanced Customer Experience: Conversational AI offers 24/7 instant support, boosting customer satisfaction and engagement through immediate responses and personalized interactions.
- Improved Efficiency: Automating routine inquiries with conversational AI frees human agents for complex tasks, streamlining workflows and increasing productivity.
- Lower Costs: Conversational AI automates customer interactions, providing a cost-efficient solution for continuous, global support and reducing expenses on staffing and training.
- Increased Accessibility: Conversational AI improves access for users with disabilities and varying technical skills through features like voice-activated assistance and multilingual support.
- Greater Productivity: Automating repetitive human tasks through conversational AI minimizes errors and lowers costs, leading to higher operational efficiency.
- Stronger Customer Engagement: Personalized experiences and round-the-clock availability offered by conversational AI enhance customer engagement and satisfaction.
- Personalized Interactions: Conversational AI uses customer data to tailor interactions, offering relevant recommendations and a more helpful experience.
- Potential Revenue Growth: Conversational AI can increase sales through effective cross-selling and upselling by providing personalized suggestions.
Conversational AI vs. Generative AI
Let’s clarify one of the most common misconceptions about conversational AI and generative AI. Although these terms are used interchangeably, they are actually quite different. Below is a comparison to highlight their key differences:
Feature | Conversational AI | Generative AI |
Primary Focus | Facilitating interactive dialogue and understanding user intent in natural language. | Creating new, original content (text, images, audio, etc.). |
Goal | To enable human-like interactions and provide helpful, contextually relevant responses. | To produce novel outputs that often mimic human creativity. |
Output | Responses within a conversation are often aimed at providing information, assistance, or completing a task. | A wide range of content, including articles, images, music, code, and more. |
Key Applications | Chatbots, virtual assistants, customer service, and voice-controlled interfaces. | Content creation, art generation, code generation, and synthetic data generation. |
Relationship to Input | Responds to and is guided by user input within a conversational context. | Can be prompted by input but aims to generate something new, not just respond. |
How Conversational AI Works: The Underlying Mechanisms
Conversational AI operates through several processes that enable it to understand, interpret, and respond to human language in a natural and contextually relevant manner. The core components of conversational AI include:
- Input Generation and Reception: The end user provides input to the conversational AI system, which can be text typed into a chat interface or a voice prompt spoken to a voice assistant. If the input is voice, speech recognition technology (like Automatic Speech Recognition—ASR) converts it into machine-readable text.
- Input Synthesis and Analysis: Once the input is received and potentially converted to text, the system uses natural language understanding (NLU) to process and analyze it. This involves understanding the meaning of the user’s words, identifying their intent, and extracting relevant information from the input.
- Dialogue Management: In this stage, the system determines the appropriate response based on the analyzed input and the current context of the conversation. NLG then formulates the response in natural language. This involves selecting the right words and phrasing to create a clear and relevant answer.
- Output Generation: The generated response is then delivered back to the user. This can be in text displayed on a screen or spoken output using text-to-speech synthesis.
- Reinforcement Learning (Continuous Improvement): Over time, machine learning algorithms refine the system’s responses based on user feedback and ongoing interactions. This process, often involving reinforcement learning, helps the conversational AI become more accurate, relevant, and effective in its interactions.
Examples and Use Cases of Conversational AI
Conversational AI has found applications across various industries and use cases, transforming how businesses interact with customers and employees perform their tasks. Here are some key use cases:
- Customer Service and Support: This is one of the most common areas for conversational AI.
- Chatbots on websites and apps provide 24/7 first-line support, answering frequently asked questions (FAQs) about shipping, products, services, and more. They can troubleshoot issues and offer self-service options outside regular business hours.
- AI agents can handle more complex inquiries and resolve issues autonomously, learning and adapting over time. They offer personalized recommendations based on past interactions and customer data during the shopping experience.
- Virtual assistants can guide customers, provide information, and even facilitate transactions, improving customer satisfaction and engagement.
- Conversational AI can be integrated across multiple channels, including social media and messaging apps, to provide consistent support.
- Employee Support and Productivity: Conversational AI is increasingly being used to assist employees within organizations.
- HR chatbots can help with employee training and onboarding, answer questions about benefits and policies, and update employee information.
- IT help desks can utilize AI agents to walk employees through troubleshooting steps for common technical issues and escalate complex problems to human agents with relevant context.
- Sales and Commerce: Conversational AI can improve the shopping experience and drive sales.
- Conversational commerce lets customers place orders, book tickets, or make reservations through natural language interactions.
- AI agents on e-commerce platforms can answer questions about sizing and material, recommend similar styles, and even offer discount codes, streamlining the shopping experience.
- They can also assist in creating digital storefronts, automating tasks like populating product catalogs and writing product descriptions.
- Accessibility: Conversational AI is crucial in improving accessibility for various user groups.
- Voice-activated assistants enable individuals with visual impairments or mobility challenges to interact with devices and applications using natural speech.
- Text-to-speech and speech-to-text software facilitate communication and interaction for individuals with different needs.
- Language translation features can break down communication barriers and support multilingual interactions.
- Information and Search: Conversational AI can provide quick and relevant information.
- AI-powered search engine assistants (like Google Gemini and Microsoft Copilot) can quickly produce search results that best match a user’s query.
- Virtual assistants (Siri, Alexa, Google Assistant) can answer user queries with real-time information on various topics.
- Conversational Business Intelligence (BI) apps allow users to interact with data through natural language and receive outputs through data visualizations and explanations.
- Specific Industries: Conversational AI is being adopted across various sectors with tailored use cases.
- Healthcare: AI can help patients describe conditions, schedule appointments, and answer basic medical questions.
- Banking and Finance: Chatbots can handle routine banking tasks like checking balances and transferring funds.
- Internet of Things (IoT): Conversational AI is integrated into smart home devices and wearables, enabling voice control and interaction.
Risks and Challenges of Conversational AI
While conversational AI offers numerous benefits, it also presents several risks and challenges that organizations need to be aware of and address. These include:
- Potential for Biased or Offensive Outputs: Conversational AI models are trained on large amounts of data, and if this data contains biases, the AI system may inadvertently generate biased or even offensive responses. Ensuring diverse and representative training data is crucial to mitigate this risk.
- Difficulty Handling Complex Edge Cases: While conversational AI excels at handling routine inquiries, it can struggle with unusual or complex situations that deviate from its training data or predefined scripts. In such cases, seamless handover to human agents is essential to avoid frustrating users.
- Lack of Human Qualities like Empathy: Conversational AI, at its current stage, lacks genuine empathy and emotional intelligence. This is a great challenge in situations requiring understanding and responding to user emotions or nuanced tones like sarcasm. Future advancements aim to improve emotional intelligence in AI.
- Privacy and Security Concerns: Conversational AI systems collect and process user data, raising privacy and security concerns. Organizations must implement robust security measures to protect user data and comply with relevant regulations. Users may also be apprehensive about sharing sensitive information with a machine.
- Language Input Challenges: Conversational AI can struggle to understand variations in human language, including slang, jargon, regional dialects, accents, and background noise. The human factor in language, such as emotions and tone, can also be challenging to interpret accurately. While multilingual capabilities are improving, many models have been primarily trained in English.
- Implementation and Maintenance Costs and Complexity: Developing and deploying advanced conversational AI systems can demand substantial resources, including technical expertise, time, and budget. Additionally, integrating AI with existing systems and continually training and updating the models presents persistent challenges. Open-source platforms like Rasa offer customization but require coding expertise.
- Ethical Considerations and Societal Impact: The increasing use of conversational AI raises broader ethical considerations, including the potential for job displacement due to automation. Organizations should consider the societal impact of their AI implementations.
Conversational AI Platforms and Implementation
Implementing Conversational AI involves selecting appropriate platforms and following a structured development process to create effective and efficient AI-driven communication systems. Below is an outline of some Conversational AI platforms:
- Google Dialogflow: A comprehensive platform to design and integrate conversational user interfaces into mobile apps, web applications, devices, and bots. It supports voice and text-based interactions and integrates with various messaging platforms.
- IBM Watson Assistant: Provides AI-powered virtual agents capable of engaging in humanized conversations. It offers features like intent recognition, entity extraction, and dialog management, facilitating deployment across multiple channels.
- Microsoft Bot Framework: A comprehensive framework for building enterprise-grade conversational AI experiences. It supports multiple programming languages and enables integration with various channels, including Microsoft Teams and Slack.
- Amazon Lex: A service for building conversational interfaces using voice and text. It uses the same technology that powers Amazon Alexa, providing advanced deep learning functionalities for automatic speech recognition and natural language understanding.
- Kore.ai: An enterprise-grade platform offering tools to design, build, test, and deploy AI-rich chatbots and virtual assistants across various channels. It provides pre-built templates and supports complex workflows.
- LivePerson: AI-powered conversational solutions that enhance customer engagement. It offers tools for creating bots that can interact across messaging channels, websites, and mobile apps.
- Verint: Provides conversational AI solutions focused on customer engagement, offering capabilities like voice self-service, chatbots, and intelligent virtual assistants to improve customer interactions.
- Rasa: An open-source framework for building AI assistants, allowing developers to create customizable and self-hosted chatbots. It offers flexibility and control over conversational experiences.
- OpenAI GPT: A language model capable of generating human-like text, useful for creating conversational agents that require advanced language understanding and generation capabilities.
- NVIDIA Riva: A GPU-accelerated SDK for building and deploying fully customizable, real-time conversational AI applications, offering high-performance speech and translation capabilities.
- Zendesk AI Agents: Integrates AI into customer service platforms to automate responses, route inquiries, and provide support, enhancing customer experience and operational efficiency.
General Steps for Implementing a Conversational AI Application
Implementing a conversational AI application involves a structured approach to ensure it meets the intended goals and provides a positive user experience. Here are general steps to consider:
- Define Goals and Use Case: Clearly establish the objectives you want to achieve with the conversational AI and identify the specific problems you want to solve. Be specific about what customer service KPIs or employee support goals you aim to improve. Determine the scope and purpose of the tool.
- Identify Potential User Inputs and Needs: Compile a list of frequently asked questions (FAQs) or anticipate the types of queries end-users will likely make. Analyze existing customer interaction data or feedback to understand user needs and pain points.
- Design the Conversation Flow and Scope: Based on the identified inputs and goals, design the dialogue or conversation flows the AI will follow. Define the intents (goals the user expresses) and relevant entities (nouns or keywords related to the intents). Determine the level of complexity the AI needs to handle.
- Choose the Right Conversational AI Platform or Tools: Select a platform or set of tools that aligns with your specific use case, technical expertise, budget, and integration requirements. Consider factors like ease of implementation, NLP capabilities, pre-built integrations, customization options, scalability, security, and vendor support. Options range from no-code platforms to open-source frameworks.
- Prepare and Integrate Data Sources: Identify and integrate the knowledge bases or data sources the AI will need to access to provide accurate and relevant responses. This might include FAQs, product information, internal documentation, or CRM data. Ensure the AI can access and synthesize information from these sources.
- Build and Train the AI Model: Develop the conversational AI model using the chosen platform or tools. If applicable, this involves training the model on relevant data, including text and speech. Utilize techniques like fine-tuning to tailor the system to your organization’s needs. For more advanced systems, reinforcement learning can improve accuracy over time.
- Design the User Interface and Experience: Consider the various mediums through which users will interact with the AI (text, voice, etc.) and design an intuitive and user-friendly experience. Ensure the AI’s personality and tone align with your brand.
- Develop and Test a Prototype: Build a prototype of the conversational AI application to test its functionality and identify potential issues. Conduct internal testing to ensure the AI handles anticipated user inputs correctly and provides appropriate responses.
- Test with End Users: Once the prototype is functional, conduct testing with real end users to gather feedback on its usability, accuracy, and overall experience. Use this feedback to identify areas for improvement and refinement.
- Iterate and Refine: Based on the testing results, iterate on the design, training data, and conversation flows to improve the AI’s performance and address any identified issues. Continuously monitor and analyze the AI’s interactions to identify areas for ongoing optimization.
- Implement and Deploy: Once the conversational AI application meets the desired performance standards, deploy it on the chosen channels (website, app, etc.). Ensure seamless integration with existing customer support software or other relevant systems.
- Monitor Performance and Gather Feedback: Continuously monitor the AI’s performance using relevant metrics after deployment. Collect customer feedback through surveys or other means to understand user satisfaction and identify areas for further improvement. Quality assurance tools can also help evaluate interactions.
- Maintain and Update: Conversational AI models require ongoing maintenance and updates to improve accuracy, expand knowledge, and address new user needs or evolving language. Continuously train the model with new data and refine the conversation flows as needed.
Conclusion
Conversational AI significantly impacts how we interact with technology, moving beyond pre-programmed commands to more natural and human-like dialogues. It uses NLP and ML for human-like dialogue, offering benefits like improved customer experience and operational efficiency. While powerful, it presents challenges such as bias and handling complex situations. Implementing conversational AI involves steps from defining goals to continuous monitoring. The future promises even more intelligent and human-like interactions.
Further Resources
- AI Agents: An Overview of Types, Benefits, and Challenges
- What is Model Context Protocol: Everything You Need to Know About the MCP
- Agentic Object Detection: The Future of Image Recognition
- Vector Quantization in the Age of Generative AI
- Zero-Shot Learning: How AI Learns Without Examples
- Chain-of-Thought Prompting: Enhancing LLM Reasoning
- CNNs with Self-Supervised Learning: A New Paradigm for Computer Vision
Frequently Asked Questions
Q 1. What is a conversational AI?
Conversational AI refers to technologies that enable machines to understand and respond to human language, simulating natural conversation.
Q 2. What is the difference between chatbot and conversational AI?
A chatbot is a specific application of Conversational AI, typically designed for specific tasks. Conversational AI is the broader technology that powers these chatbots and other interactive systems.
Q 3. Which is the best conversational AI?
“Best” depends on the specific use case. Leading platforms include Google Cloud’s Dialogflow, IBM watsonx, and Amazon Lex, each with strengths in different areas. Open Source options like Rasa are also very powerful.
Q 4. How to create a conversational AI?
Creating a Conversational AI involves defining goals, designing dialogue flows, training models with data, and integrating with existing systems, often using platforms like Dialogflow or Rasa.
Q 5. Is ChatGPT a conversational AI?
Yes, ChatGPT is a form of Conversational AI, specifically a large language model designed for generating human-like text in response to prompts, and engaging in dialogue.